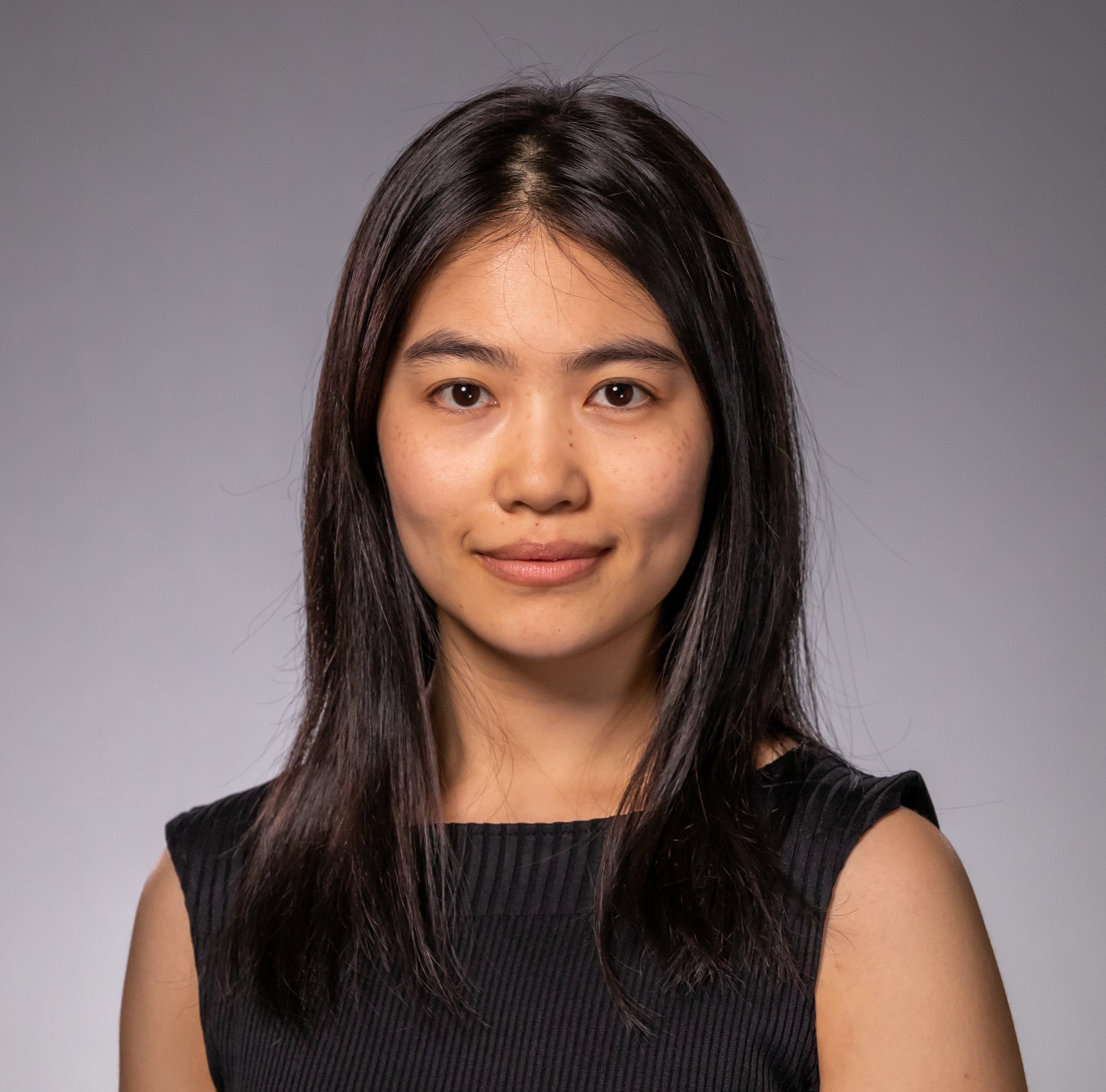
詹妮 About
I am a postdoc at Princeton University, advised by Ryan Adams, working on artificial intelligence for design and simulation of dynamic processes and materials. I completed my PhD at Carnegie Mellon University, advised by John Kitchin, with dissertation titled "Machine learning models and uncertainty for atomic simulations". My published research includes fast, accurate models to approximate quantum chemical methods, investigation of transport phenomena and dynamics of liquid alloys, uncertainty quantification for machine learning and physical properties, and graphical and probabilistic methods for finance and macroeconomics.
While at CMU, I also received an MS in machine learning and interned at JPMorgan Chase, working on strategy at the Treasury and Chief Investment Office. Previously, I worked as a chemical engineer and graduated magna cum laude with a BS in chemical engineering from the University of Texas at Austin.
Publications
-
Model-Specific to Model-General Uncertainty for Physical Properties [doi] [github] [graphical abstract]
Ni Zhan, John R. Kitchin
Industrial & Engineering Chemistry Research 2021
- Machine Learning Models and Uncertainty for Atomic Simulations [PDF] [recording]
Ni Zhan
PhD Dissertation 2021
Thesis Committee: John Kitchin, Zachary Ulissi, Michael Widom, Aditya Khair, Erik Ydstie -
Origin of the Stokes-Einstein Deviation in Liquid Al-Si [doi] [code] [simulation preview]
Ni Zhan, John R. Kitchin
Molecular Simulation 2021
Presented talk at American Chemical Society Spring Meeting 2021 -
Uncertainty quantification in machine learning and nonlinear least squares regression models [doi] [github]
Ni Zhan, John R. Kitchin
Aiche Journal 2021
Presented two talks at Aiche Annual Meeting 2019 and Machine Learning in Science and Engineering Conference 2019 -
Where does the Stimulus go? Deep Generative Model for Commercial Banking Deposits [arXiv] [poster]
Ni Zhan
Neurips Workshop on ML for Economic Policy 2020 -
Graphical models for financial time series and portfolio selection [doi] [graphical abstract]
Ni Zhan, Yijia Sun, Aman Jakhar, He Liu
Proceedings of International Conference on AI in Finance 2020
Presented two talks at International Conference on AI in Finance, 2020 and Toronto Machine Learning Society, 2021
-
Modeling Superalloys using Machine Learned Potential [poster]
John Kitchin, Jenny Zhan, Michael Widom, Bojun Feng, Jim Lill, Chris Woodward
Published as Chapter in PhD Dissertation
Presented talk at Department of Defense High Performance Computing User Group Meeting, 2019
2021
2020
2019
Projects
- Recommender Systems and Statistical Guarantee for Collaborative Filtering [PDF]
Ni Zhan, Yilin Yang, Zicheng Cai
CMU 10716 Advanced ML: Theory and Methods, Spring 2021
Project summarized statistical guarantees of matrix completion problem, following matrix incoherence property (Candes and Recht 2009) and spikiness and rank measures (Negahban and Wainwright 2012). We additionally summarized the extension of the latter to a graph regularized matrix completion, as shown by Rao et al. 2015. -
Music Recommender System and Image Dimensionality Reduction [PDF]
Yilin Yang, Ni Zhan
CMU 10805 ML with Large Datasets (Distributed Computing), Fall 2020
Music recommender on million songs using collaborative filtering, Spark on AWS Elastic Map Reduce. -
Graph Convolutional Neural Network for Atomic Structures [PDF] [graphical abstract]
Junwoong Yoon, Ni Zhan, Mingjie Liu
CMU 10707 Deep Learning, Spring 2019
-
Identifying Duplicate Questions using Siamese LSTM Architecture [PDF] [graphical abstract]
Junwoong Yoon, Ni Zhan
CMU 10701 Graduate Introduction to Machine Learning, Fall 2018